Keynotes
Fortifying Your Defenses: Techniques to Thwart Adversarial Attacks and Boost Performance of Machine Learning-Based Intrusion Detection Systems
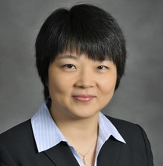
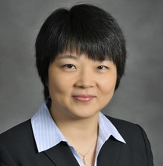
Wenjing Lou
Wenjing Lou is the W. C. English Endowed Professor of Computer Science at Virginia Tech and a Fellow of the IEEE. She holds a Ph.D. in Electrical and Computer Engineering from the University of Florida. Her research interests cover many topics in the cybersecurity field, with her current research interest focusing on wireless networks, blockchain systems, trustworthy machine learning systems, and security and privacy problems in the Internet of Things (IoT) systems. Prof. Lou is a highly cited researcher by the Web of Science Group. She received the Virginia Tech Alumni Award for Research Excellence in 2018, the highest university-level faculty research award. She received the INFOCOM Test-of-Time paper award in 2020. She is the TPC chair for IEEE INFOCOM 2019 and ACM WiSec 2020. She was the Steering Committee Chair for IEEE CNS conference from 2013 to 2020. She is currently a steering committee member of IEEE INFOCOM and IEEE CNS. She served as a program director at US National Science Foundation (NSF) from 2014 to 2017.
Secure Wireless Agile Networks (SWAN): Novel ML Applications in IoT Intrusion Detection and Secure RF Design
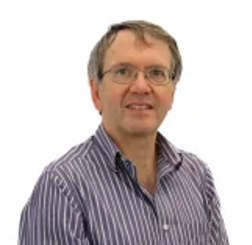
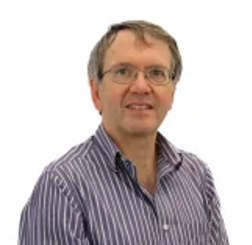
Mark Beach
Professor Mark Beach (CEng, MIET, SMIEE) has over 35 years of experience in physical layer wireless research including spread spectrum; adaptive and smart antennas for capacity and range extension in wireless networks; MIMO aided connectivity for through-put and spectrum efficiency enhancement; millimetre wave technology, as well as secure, robust and frequency agile radio frequency technologies. He leads the delivery of the UKRI/EPSRC SWAN Prosperity Partnership in Secure Wireless Agile Networks, Expert Panel Member to DCMS on 6G, is a Co-Director of CDT in Communications, and also the School Research Impact Director. Mark is a co-founder of the Cambridge based company, ForeFrontRF, creating frequency agile technology to replace fixed frequency SAW and BAR components commonplace in cellular phone technology.